Retinal image analysis by mixture model based clustering and discriminant analysis for automatic detection of hard exudates and haemorhages: A tool for diabetic retinopathy screening
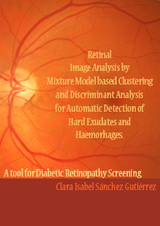
C. Sánchez
- Promotor: R. Hornero
- Graduation year: 2008
- Universidad de Valladolid, Spain
Abstract
Diabetic Retinopathy (DR) is a disorder of the retinal vasculature that eventually develops to some degree in nearly all patients with diabetes. The growing incidence of diabetes has caused DR to become the major cause of blindness and vision defects in developed countries. In view of the increasing prevalence of diabetes mellitus and DR throughout most of the world, mass screening is time consuming and requires many trained graders to examine the fundus photographs searching for retinal lesions. A reliable method for automated assessment of the presence of lesions in fundus images would be a valuable tool in assisting the limited number of professional and reducing the examination time. Additionally, this system could be a useful clinical aid in the routine eye care by displaying retinal images with enhanced details and facilitating diagnosis. This thesis aims at developing a Computer-Aided Diagnosis (CAD) technology to assist ophthalmologists in DR screening programs, in order to detect and quantify early signs associated with DR in fundus photographs. In the onset of the disease, several visual retinal abnormalities appear in the retinal fundus, including red lesions, such as microaneurysms and haemorrhages, and white lesions, such as hard exudates and cotton-wool spots. Among these lesions, haemorrhages and hard exudates are the most prevalent in background retinopathy Therefore, their detection is critical for DR mass screening. Additionally, hard exudates represent the most specific maker for the presence of coexistent retinal oedema, the major cause of visual loss in the nonproliferative forms of DR. The principal aim of this work is to develop novel computer methods to automatically detect and quantify haemorrhages and hard exudates in retinal images as an aid for the DR diagnosis in screening programs. As a first step, several methods are studied for the automatic detection of anatomical structures: the optic disk, the blood vessels and the posterior pole. The detection of these features provides a retinal coordinate system for retinopathy grading. Besides, their localization is indispensable to reduce the number of false detections due to their similarities to retinal signs in terms of brightness and contrast. Different image analysis techniques, such as wavelets, mathematical morphology and Expectation Maximization algorithm, are investigated to address the localisation of the optic disk, blood vessels and the vascular arcades. The automatic detection of haemorrhages and hard exudates relies on an innovative initial image segmentation based on mixture model based clustering, followed by a subsequent refinement stage using supervised classification. The clustering process considers the probability distribution function of the entire image as a mixture of individual distributions. The resulting pixel-cluster memberships provide a transformation of the pixels into different image regions which are suspected of corresponding to retinal lesions. Finally, a study of different discriminant analysis techniques, such as linear discriminant analysis, quadratic discriminant analysis, logistic regression and k nearest neighbours, is performed to classify regions into haemorrhages or hard exudates. Therefore, an interpretation of the retinal image will be achieved detecting DR signs and identifying retinal images with the presence of these ocular symptoms.